In 2023, many energy companies were hesitant to adopt AI due to uncertainty about its capabilities. By 2024, this hesitation shifted to caution, as most now understand AI's vast potential. It’s now easier to imagine AI causing complications than unlocking value because of concerns around regulation compliance, data security, complexity, or uncertain ROI.
However, the energy industry is transforming, driven by the urgent need for sustainability, efficiency, and resilience, and AI is at the forefront of this revolution. AI is a powerful tool that holds the potential to revolutionize how energy is produced, distributed, and consumed. From optimizing grid management to predictive maintenance and enhancing customer engagement, AI offers many opportunities to drive innovation and operational excellence.
Here we’ll explore a few ways AI can drive value in the energy industry and how your company can take the first steps toward realizing that value quickly and with confidence.
Join us for our "Scaling AI: Transforming the Oil & Gas Industry with AI Solutions" webinar on November 12!
AI use cases in the energy industry
Increasing daily efficiency
AI can make your team more efficient by automating routine tasks and providing insights to streamline meetings. For example, AI tools can transcribe meetings, summarize key points, and suggest action items, freeing up time for strategic activities. Natural language processing (NLP) and machine learning (ML) can automate these processes and analyze meeting data for improvements.
Reducing the cost of serving customers in a highly regulated environment
AI can navigate the regulatory complexities of the energy industry by automating compliance checks and optimizing customer service processes, ensuring adherence to regulations and reducing the risk of non-compliance costs.
Increasing time-to-revenue for complex energy solutions
AI can streamline the energy sector’s complex and time-consuming revenue models. For example, in the oil and gas industry, AI can accelerate the drilling application process by automating data entry that historically has been manual, reducing error and rework. For utilities, AI can recommend intelligent configure/price/quote (CPQ) scenarios based on customer buying patterns.
Enhancing operational planning
AI can model and predict supply-and-demand scenarios, helping energy leaders make informed decisions about resource allocation, production schedules, and inventory management. These solutions are particularly useful for field crew optimizations when asset utilization and cost optimization are key.
Credera’s approach to getting started
While the journey to AI adoption is often slowed by internal challenges and the daunting task of knowing how to start safely, choosing the right use cases and the right approach can address those challenges. As you begin to explore those opportunities, you might think of your company’s relationship with AI as being similar to a personal relationship: Confidence and trust are built incrementally through deliberate investments of time and resources.
We help our clients begin leveraging AI through quick, incremental projects, allowing them to test and learn while mitigating risk. The primary benefits of this approach include:
Gaining hands-on experience: Start with small projects to build familiarity with AI tools and techniques.
Addressing data security concerns: Ensure that your AI initiatives comply with data privacy regulations and best practices.
Demonstrating value: Show quick wins to build momentum and support for further AI investments.
Credera’s recommended process: Scaling AI framework
A structured, iterative process is the key to confidently adopting AI, mitigating risks, and continuously improving your AI initiatives. Credera’s Scaling AI framework ensures your AI solutions are effective and aligned with your business objectives. It lets you build the capabilities and the guardrails, allowing you to realize business value while you're still learning.
A sandbox, a contained virtual environment, is a safe way to test and learn without impacting live networks or systems. Leveraging a sandbox and an iterative process, you can launch small projects safely, incorporate feedback from each cycle, and evolve the requirements to continuously refine and improve.
Here is a high-level look at the process we recommend:
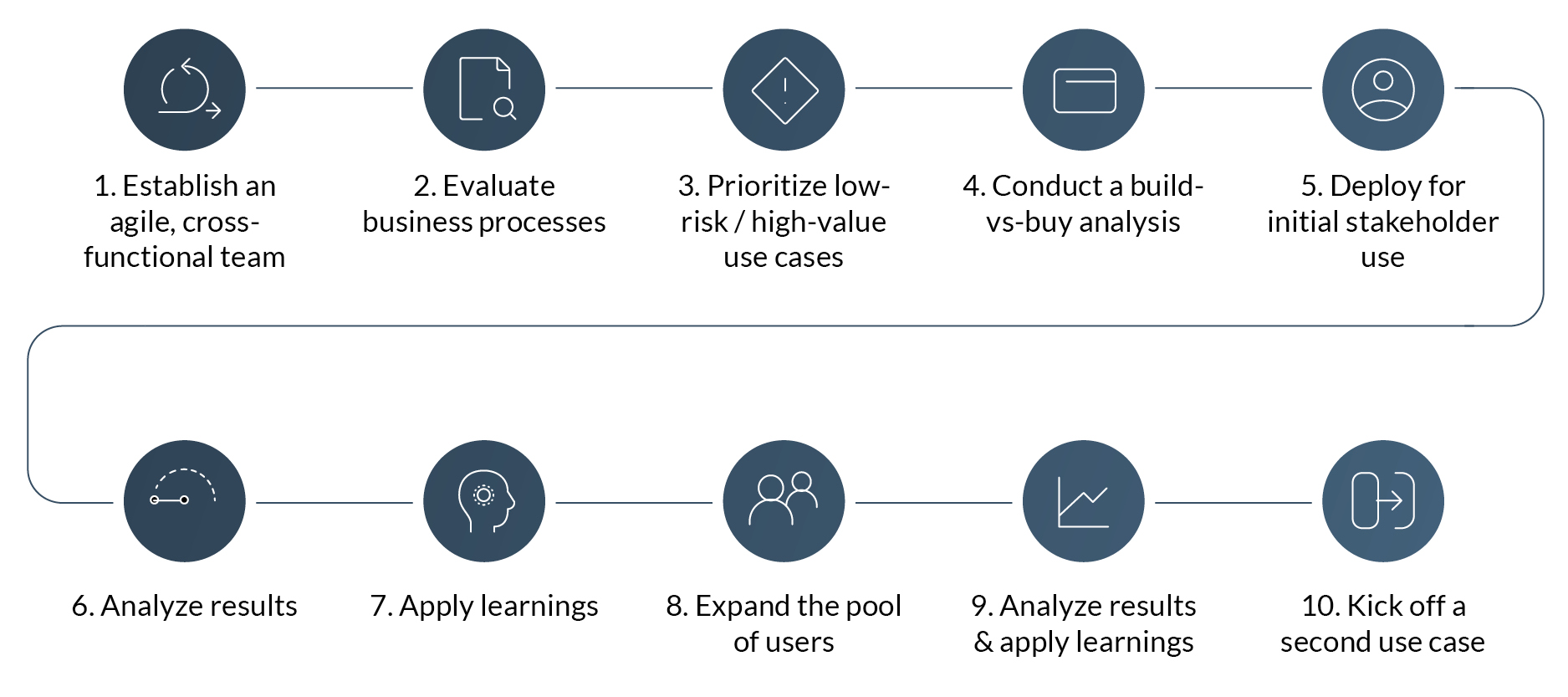
1. Establish an agile, cross-functional team
The foundation of any successful AI initiative is a strong team capable of developing and implementing AI solutions. Leveraging the varied expertise of team members, energy companies can harness the power of AI to drive innovation and address industry-specific challenges.
2. Evaluate business processes
Before diving into AI implementation, it's crucial to thoroughly evaluate your existing business processes to identify inefficiencies, bottlenecks, and other areas where AI can add the most value.
Process mapping: Document current workflows to understand each step and identify areas for improvement.
Stakeholder interviews: Engage with employees and stakeholders to gather insights and pain points.
Data assessment: Evaluate the quality and availability of the data required for AI applications.
3. Prioritize low-risk / high-value use cases
In the energy industry, risk management is crucial for ensuring safe and reliable operations. Not all AI projects are created equal. For example, using AI to anticipate equipment failures is a low-risk application that can help prevent costly downtime and accidents, but using AI for automated trading in energy markets involves higher risk due to the potential for financial losses, regulatory issues, and market volatility.
Impact analysis: Assess the potential impact of each use case on business objectives.
Feasibility study: Evaluate the technical feasibility, including data availability and resource requirements.
Risk assessment: Identify potential risks and develop mitigation strategies.
4. Conduct a build-vs-buy analysis
Determining whether to develop the pilot project in-house or purchase one that's pre-built involves understanding your team members’ abilities and availability and weighing the benefits of a custom solution against the efficiency and cost-effectiveness of off-the-shelf options.
5. Deploy for initial stakeholder use
This phase is essentially a pilot project aimed at gathering initial feedback and demonstrating value.
Pilot implementation: Roll out the AI solution in a controlled environment with a limited user base.
Training and support: Provide training and support to stakeholders to ensure successful adoption.
Feedback collection: Gather feedback from users to identify issues and areas for improvement.
6. Analyze results
After the initial deployment, analyze the results to understand the impact of the AI solution.
Performance metrics: Measure key performance indicators (KPIs) to evaluate the effectiveness of the AI solution.
Data analysis: Use data analytics to identify patterns, trends, and areas for further optimization.
7. Apply learnings
Based on the analysis, apply learnings to refine and improve the AI solution.
Iterative improvements: Make necessary adjustments to the AI model, algorithms, or processes based on feedback and performance metrics.
Documentation: Document the changes and the rationale behind them to maintain a clear record of the iterative process.
8. Expand the pool of users
With the refined AI solution, expand the pool of users to further validate its effectiveness and scalability.
Gradual rollout: Gradually introduce the AI solution to more users and departments.
Ongoing support: Continue to provide support and training to new users to ensure smooth adoption.
Feedback loop: Maintain an ongoing feedback loop to capture insights from the expanded user base.
9. Analyze results & apply learnings
Repeat the analysis process to evaluate the impact of the AI solution on the broader user base and incorporate the new learnings to further refine the AI solution and prepare for wider deployment.
10. Kick off a second use case
With the success of the first use case, kick off the second use case and repeat the process.
The bottom line
At Credera, we understand the challenges and opportunities associated with AI adoption in the energy industry. Our specialists have developed repeatable, actionable plans that can help you quickly leverage the power of AI to drive efficiency and growth.
Whether you’re just getting started with AI or looking to scale your initiatives, we can provide the expertise and support you need to succeed. Schedule a call with our AI specialists to discuss navigating your AI journey with confidence and unlocking the full potential of AI in your business, and check out the rest of the content in our AI in Energy series:
Contact Us
Ready to achieve your vision? We're here to help.
We'd love to start a conversation. Fill out the form and we'll connect you with the right person.
Searching for a new career?
View job openings