Data
Dec 08, 2020
Data Quality Part 1: 4 Likely (and Common) Causes of Bad Data Quality
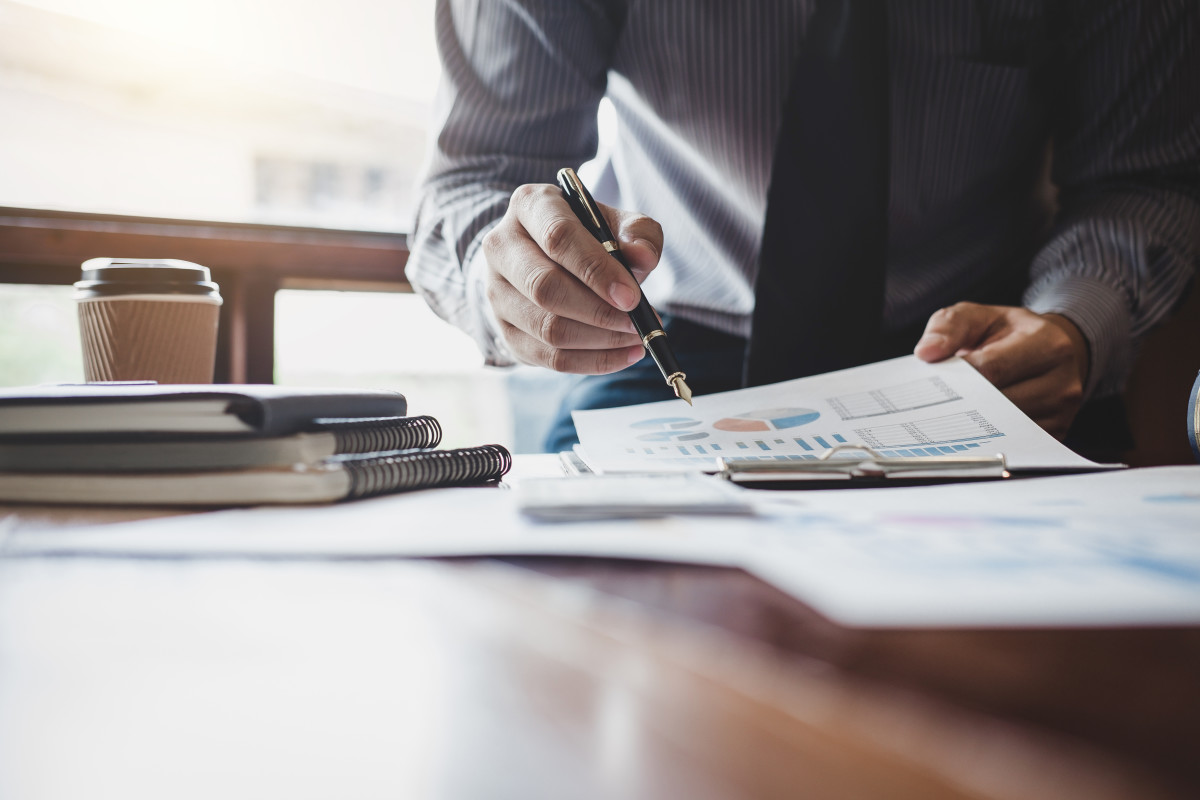
Every company, no matter the size, likely suffers from bad data and issues around data quality. While that simple fact may be fairly well understood, the reasons for these data quality issues are less well known. Based on our client work, we’ve identified the four most common issues.
Bad Data Quality Cause #1: Cracks Showing Up as Your Data Scales
While the scale of sources and the variety and volume of the data have changed radically, the core data architecture has remained largely static. Usually, a data model gets built early on, with a particular set of use cases and overall volume, but isn’t updated as the data grows, complexity of data increases, and data uses proliferate across the company. As these things grow, your data model can start to falter, which in turn produces data quality issues.
The cause is subtle and rarely noticed until it’s too late. This is partially driven by the fact that in the nascent phases of the data journey, there is relatively little data and many people. This low ratio of data to people enables them to do what humans are great at–adapting. However, unfortunately it is usually in a nonscalable way. It’s one thing to manually correct one customer record because your online form does not validate upon entry, but when you start receiving hundreds or thousands of entries daily you start feeling the pain and identify the underlying data issue.
Bad Data Quality Cause #2: Errors Propagating in Dynamic Ways
As everyone tries to move faster and control their own destiny, data evolves in unexpected ways that erode trust in underlying data and all analysis. It could be an upstream view built into a particular data source that changes for a specific set of models, or a particular data model that’s modified to accommodate an upgraded software platform that propagates the table.
Regardless of the reason, no data source exists in a bubble within a data-driven organization, and this leads to downstream data quality issues as time goes on. Errors creep in as the data evolves, and it’s often difficult to predict how they might surface in part because producers rarely know who downstream consumers are let alone how these consumers are using the data.
How often does your marketing department try some new channel or microsite Oftentimes, these are stood up very quickly and cheaply just to see if it worth the long term investment. Naturally marketing knows what they’ve done and can adapt their own metrics and dashboards to account for this experiment. However, what happens when they do a load test on the new microsite and the downstream e-commerce or supply chain team see a huge spike in traffic and aren’t aware of the test? Do they leverage all the scaling and monitoring you’ve put in place to be more nimble and respond to changes in demand? Thus, responding in short order by ramping up call center staffing, and placing larger orders with your vendors?
If so, this activity with throw off even more data that their consumers will use to make a variety of decisions. None of this could have been foreseen by that marketing team because the impacted consumers are rarely the same. The exact fluidity and organizational improvements companies have been working so hard to achieve over the past decade are the same ones that make this challenge so difficult to address.
Bad Data Quality Cause #3: Abnormalities That Aren’t Necessarily Bad Data
Classifying anomalies in data is an art, and virtually no organization has a mechanism to codify those annotations globally. Seasonality is a great example of good data that doesn’t follow a simple pattern, which can throw off models if not accounted for. If you have wild swings one year out of five, you may be dealing with accurate data that also happens to be an outlier. To handle this in isolation isn’t an issue, as a particular data point can always be disregarded or manually adjusted.
However, as time goes on, the number of metrics and sources of data often grow. Each new metric will have its own unique seasonal trends and manual overrides do not scale. The downstream models built to forecast and predict future trends are adversely affected if those values aren’t properly analyzed and managed. These new models in turn generate more inaccurate data for their consumers only reinforcing the entire cycle. Just as with cause number one, these problems usually exist from the onset of an organizations journey but are typically hidden in the early days when the people to data ratio is low.
Bad Data Quality Cause #4: Individual Needs vs. Enterprise Uniformity
Prioritization at individual levels rarely leads to globally improved data, which is exacerbated by each group using the data in a unique way. Marketing can pull data generated by the ecommerce team to develop a model for promotion schedules. However, the ecommerce team has an individual set of priorities tied to website performance, which can shift as the individual team’s priorities shift. All of this data ends up in the office of the chief financial officer, which has a team building models around financial performance tied to both marketing promotions and ecommerce trends. This data, combined with previous data sets, then ends up in human resources to predict business cycles and hiring patterns.
At each stage, the data can be modeled or re-modeled based on what that particular team needs, and in turn affect everyone else dependent on the same data for their own purposes. These changes aren’t often widely communicated, as no one is individually incented to do so. While the marketing team no doubt cares in a general sense that the e-commerce team is effective and that human resources hires the right number of people, at the end of the day, quarter, year, the only thing a marketer is measured on is marketing. Therein lies the rub, it’s unclear in a typical company who is ultimately responsible for data that each part of the business generates and refines. The business needs to remain laser-focused on running their part and they rarely have any idea who relies on their data sets. They don’t have the time or the expertise to find out.
Getting to the Root of the Data Quality Problem
Each of these issues are common across all of our customers and are something we often work to help resolve as we work with client’s individual data needs. The foundation of any good data modeling exercise begins with good data, but each one of these issues can get in the way.
What’s often the cause of these issues, and what can you do about solving them across your company’s data ecosystem? In our next blog post we discuss the typical scapegoat for data quality issues, and the real root cause of your data issues. In part three and four of our data quality series, we discuss effective data quality and how to build a system to solve your data quality issues at every level of your company. If you’re interested in having a conversation about your specific data quality issues, feel free to reach out to us at findoutmore@credera.com.
Contact Us
Ready to achieve your vision? We're here to help.
We'd love to start a conversation. Fill out the form and we'll connect you with the right person.
Searching for a new career?
View job openings